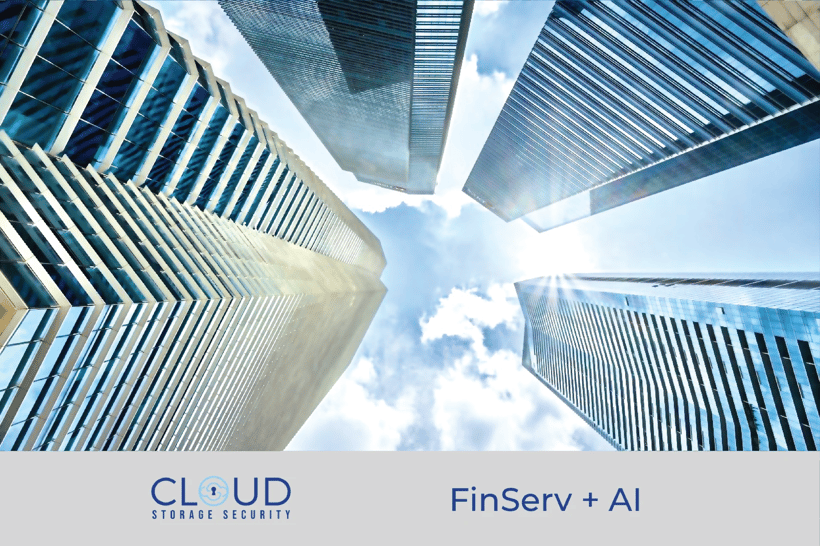
A financial services company became aware of a critical need to secure their generative artificial intelligence (GenAI) models against malware infiltration. These models are used to efficiently process large amounts of unstructured financial data to deliver rapid quantitative and qualitative analysis across multiple business lines.
Customer Challenge
This enterprise was using datasets from a leading third party machine learning (ML) model vendor to help build and train their own ML and AI models, using Amazon Simple Storage Service (Amazon S3) to store the data before using it for training purposes. Reports revealed that the model vendor unknowingly hosted hundreds of datasets that contained instances of malicious functionality thereby creating numerous security vulnerabilities and exposing users to potential threats.
Issues like this are becoming a growing concern among organizations that harness third party data when building GenAI applications. Through external data, malicious code can enter their environment; leveraging external data sets exacerbates the risk by adding an additional point of entry for malware.
This, combined with concerns about inadvertently sharing sensitive information through the text outputs of their application, drove the company to seek a solution that allows for continued AI and ML research while ensuring effective security and stability.
Overview of Customer Needs:
- Malware Detection - ML models present unique security issues. Malicious code can exist within the models, jeopardizing the security of the business if a payload is activated when the data is used. Potentially malicious files, while dormant in cloud storage like Amazon S3, pose significant downstream risks when used across the various lines of business.
- Privacy Compliance - The customer has strict data privacy practices and cannot allow solutions to create opportunities for security breaches. This means any solution is required to launch as a super-private deployment that is compliant with internal private architecture requirements that cut off public access, and deliver an air gapped system.
- Sensitive Data Scanning - The company’s application output needs to be properly scanned and vetted for sensitive data before it is delivered. They needed a solution that scans and flags data containing personal user information, private financial data, and critical trades and insights without delaying the delivery of approved information to the end user.
GenAI Secure Implementation and Results
As a safeguard, the company integrated GenAI Secure by Cloud Storage Security into their input pipeline to scan the model data before it is used by their GenAI application. In doing so, the company uploads the data to an Amazon S3 staging bucket and GenAI Secure automatically initiates an event-based malware scan. When the scan completes, malicious data is moved to an S3 quarantine bucket while clean data is moved to a S3 custom model bucket, which is used for training the FMs.
The Result: Secure Model Data - GenAI Secure met critical security requirements to manage threats related to the ML models used in the company’s GenAI application.
- This implementation of GenAI Secure quarantined numerous suspicious files, thereby protecting the business and downstream users from risk.
- Quarantining high-risk files prevented the transmission of potentially malicious data.
Real time assessment capability was leveraged to prevent threats coming from models escaping to downstream applications and protected the enterprise from malicious data stored in AWS related to ML/AI models. The enterprise’s CIO was pleased to find their ML/AI models and their data to be secure, eliminating numerous threats that could have resulted in security breaches across the organization.
The Result: Malware-free Storage - Working automatically as data enters and exits AWS, GenAI Secure effectively manages the review and flagging of ML/AI data so that data teams can be confident in the security of their AWS storage.
- Automatic scanning enables continuous monitoring.
- Flagging of files allows data teams high-visibility to active threats.
Inadvertently sharing sensitive information is harmful to any organization. In the financial space, such data leakage is disastrous. Compliance with financial regulations and privacy is paramount. As more companies in the financial services space adopt AI models and implement AI-based technologies, ensuring individually identifiable information is secure becomes critical. This is especially true when a FinServ company is using an AI chatbot.
In this case, the company implemented GenAI Secure to also scan the chat output. Chat output is sent to an Amazon S3 output bucket before it’s delivered to the end user. GenAI Secure automatically kicks off a Lambda function to make HTTP POST calls to an Application Load Balancer, which distributes data across multiple API endpoints that automatically scale for sensitive data scanning. When the scan is complete, the API endpoint uploads non-sensitive data to an S3 application bucket, which is used to feed the downstream GenAI chatbot. As a result, only publicly available information is distributed to the end user, while the AI application may have access to more sensitive information for other internal applications. This specific case applies across industries, whether for a financial services company, healthcare organization, or public sector business, unchecked AI chatbots can create cascading challenges.
The Result: Privacy Assurance - GenAI Secure combs data securely, separating out sensitive data, without the data ever leaving the enterprise’s environment.
- Thoroughly scanning data for malware prevented a data breach.
- Scanning for PII ensured the protection of sensitive customer data.
Moreover, the enterprise was able to install GenAI Secure within their AWS environment in order to meet their security and privacy requirements, which included restricting public internet access as part of a private deployment.
The Result: Operational Efficiency - Solutions should simplify the challenges faced, and GenAI Secure does just that.
- The company subscribed to GenAI Secure in AWS Marketplace and was able to install and deploy it within their AWS account in a rapid timeframe.
- Leveraging CSS’s easy to understand dashboards, the enterprise was able to easily scan and view potential threats.
- CSS saved the enterprise time and budget, freeing up IT resources to focus on developing new solutions rather than managing the endless malware threat.
Conclusion
It’s crucial to identify potential security threats and implement proactive cybersecurity measures— especially within GenAI and machine learning ecosystems. By directly addressing the concerns of both malware detection and data privacy, GenAI Secure equipped this financial services company with a strong defense against evolving threats that ensures the integrity and security of their diversifying AI/ML models.
Moving Forward with CSS
Contact us to schedule a short demo to learn more about how Cloud Storage Security can help your business understand and achieve data integrity for its GenAI applications.